The emergence of large language models (LLMs) has sparked a growing interest in journalism due to their accessibility and ease of use. Although these technologies are not without their flaws and raise many ethical issues (bias in training data, lack of reliability, copyrighted data, risk of plagiarism, generation of artificial hallucinations, problems with logical reasoning, failure to understand concepts and contexts in a human sense, etc.), they are the subject of much experimentation in newsrooms, where they are seen as having promising potential to support and augment journalistic tasks. However, they require human supervision to ensure the accuracy and reliability of their results. This article presents twenty possible types of tasks. The examples accompanying the descriptions were partly generated (under human supervision) by ChatGPT.
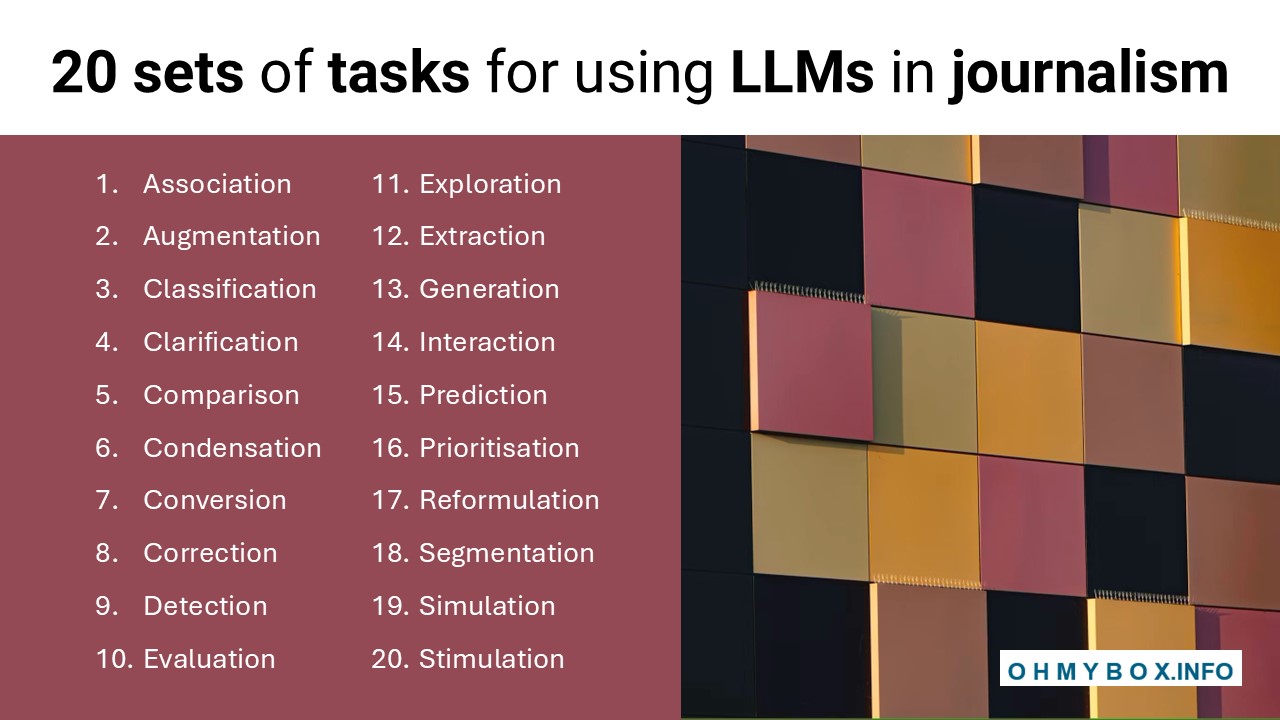
1. Association
Description: Associate concepts or ideas from texts to make new connections, link data sets to complete, explain or correlate, etc.
Example: Linking together several seemingly unrelated events or news items to provide an in-depth analysis of a geopolitical trend.
2. Augmentation
Description: Enrich existing data or content with additional information to deepen understanding or explore new angles.
Example: Enrich a news article about climate change with additional data from new studies or recent statistics.
3. Classification
Description: Organise data into pre-defined groups to identify and analyse specific categories.
Example: Organise documents or data by subject (politics, economics, sport, culture) to make them easier to analyse.
4. Clarification
Description: Simplify complex concepts to make them more understandable and accessible.
Example: Explain the results of statistical indicators such as p-values in clear, accessible language so that their meaning and significance can be better understood.
5. Comparison
Description: Analyse the similarities and differences between two or more texts, ideas or sets of data.
Example: Compare the environmental policies of different countries in an analytical article to highlight their respective strengths and weaknesses.
6. Condensation
Description: To summarise a text or set of documents to produce a shorter, more concise version.
Example: Summary of a 200 pages report.
7. Conversion
Description: Translate documents or adapt the language to make them easier to understand.
Example: Translating an interview given by a political leader in Spanish into English to make it accessible to an English-speaking audience.
8. Correction
Description: Improve the grammar, spelling and style of a text to improve the quality of writing.
Example: Correct spelling and grammatical errors in an article before it is published.
9. Detection
Description: Identify patterns or anomalies in data sets to identify significant trends or irregularities.
Example: Identifying emerging trends in a new government’s budget data.
10. Evaluation
Description: Analyse the reliability of information, its value or tone and the emotions expressed.
Example: Analysis of emotions in a corpus extracted from social networks (positive, negative, fear, etc.).
11. Exploration
Description: Exploring different angles or perspectives on an issue, proposing concepts, strategies or lines of thought.
Example: Suggest different political, social and economic perspectives to cover a complex topic from different angles.
12. Extraction
Description: Identify and classify key elements in a text (names, places, dates, events).
Example: Extracting key information from a long political speech, extracting names of people from documents.
13. Generation
Description: Create content from prompts, whether for text, dialogue or scripts.
Example: Generate a first draft of an article based on a set of facts, which the journalist can then refine and enrich before publication.
14. Interaction
Description: Taking part in dialogues, answering questions or providing conversation support.
Example: Using a chatbot integrated into a news site to answer readers’ questions in real-time.
15. Prediction
Description: Predicting future outcomes or behaviour based on existing data.
Example: Predicting the results of the next election based on analysis of polls and voting trends from previous elections.
16. Prioritisation
Description: Prioritise ideas or information according to their relevance or feasibility.
Example: Suggest a hierarchy of headings on a web or printed page.
17. Reformulation
Description: Transforming a text while retaining its meaning by changing tone, structure or perspective.
Example: Reformulating a quote from a politician to make it more understandable without changing the original meaning.
18. Segmentation
Description: To divide a text into different parts or sections for better organisation (paragraphs, chapters, thematic sections).
Example: Dividing a long document into several sections to make it easier to explore or summarise.
19. Simulation
Description: Reproduce hypothetical or predictive scenarios to test ideas or explore potential outcomes.
Example: Simulate the impact of a hypothetical economic policy on employment or the cost of living to illustrate a future article.
20. Stimulation
Description: Inspire and encourage creative thinking to help generate ideas.
Example: Use prompts to stimulate ideas for original or unpublished articles on a topic or event.