Presented at the 5th International Press Freedom Seminar: Artificial Intelligence & Journalism, UGent, December 13, 2023
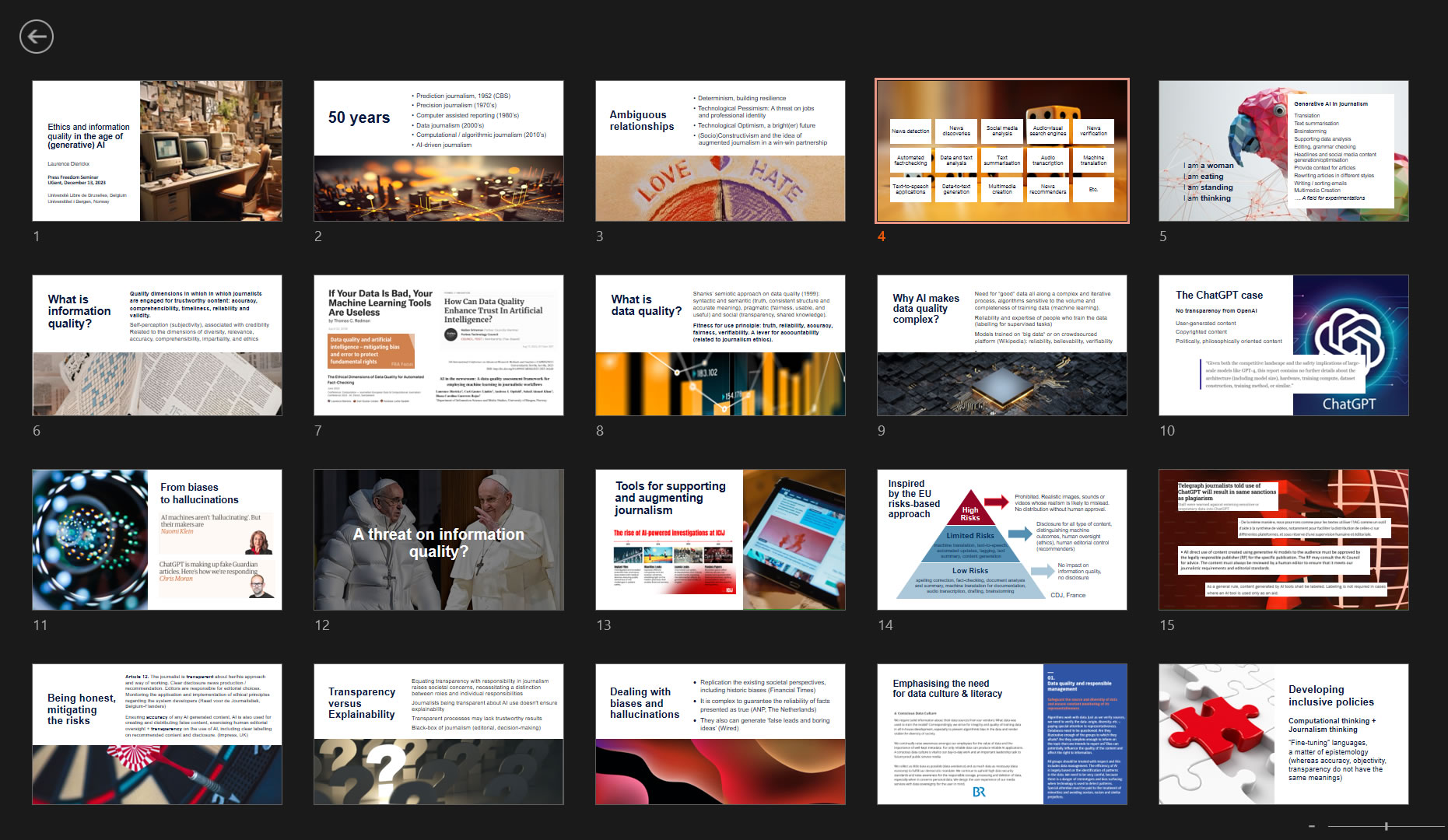
1.
Ethics and information quality in the age of (generative) AI
2.
Computers have been part of the history of journalism for more than 50 years. The first experiment took place in the USA in 1952. CBS News used a Remington Rand Univac to predict the outcome of the Eisenhower-Stevenson presidential election. The machine made no mistake. Eisenhower won. It had a one-in-two chance of being wrong. The power of computing and the ability to store and process increasing amounts of data led some pioneers, particularly in the US, to take advantage of these new opportunities. Their main focus was using data to understand society better and develop innovative investigative methods. These first experiences of data-driven journalism were considered elite and led to many Pulitzer Prizes.
Today, we can consider working with data as a specialised journalism area requiring specific professional skills. At the same time, journalism students are still reluctant to work with numbers, even though numbers have always been a part of journalism. Also, there are a lot of humans and social realities beyond all of these numbers. Furthermore, the development of AI technologies highlights the need for robust AI literacy, not only to use AI systems within newsrooms but also to be able to investigate algorithmic-driven societies. And it all starts with data.
3.
In this long history, more than 50 years already, journalists have always had an ambiguous relationship with technology. And there is no exception with AI. Determinism corresponds to building resilience. Technological developments are considered unavoidable and have benefited from creating the conditions for specific work and reinforcing existing professional standards.
According to technological pessimism, technological developments diverge from established professional norms and values and are not without risks of disastrous consequences for journalism. It is related to the fear of being more and more under pressure, the fear of losing jobs or even the fear of losing one’s professional identity.
On the contrary, technological optimism tackles technological advancements to catalyse the reshaping and revitalising of journalism practices. In other words, technology will save journalism. Finally, the socio-constructivism approaches are related to augmented journalism, where technology is a dynamic force that shapes journalism within its social and cultural environment.
4.
Before going further, it is essential to frame what artificial intelligence is and what it means for journalism. AI refers to computer systems that can perform tasks that mimic human intelligence. AI systems use algorithms and computational models to process large amounts of data and identify underlying patterns. As one of my colleagues said at the University of Bergen, we all acknowledge that artificial sugar is not sugar. We would recognise the same with AI systems. They relate to a wide range of technical and real-life applications. In journalism, they are used for news gathering, production, and distribution. Overall, AI algorithms are used for classification, prediction and language understanding. They are based on mathematical principles and probability. Imagine you have a standard six-sided dice. Each face of the dice is numbered from 1 to 6. When you roll the dice, there are six possible outcomes, each with an equal chance of occurring. The probability of rolling out a three is 1 out of 6 possible results. It is schematised, but it is how AI systems work. And it is all about data processing and maths, allowing machines to learn, adapt and perform complex functions.
5.
Generative AI systems are more complex, relying on neural networks and natural language processing. Large language models, such as ChatGPT, are designed to predict the probability of the next word or phrase in a given text based on statistical patterns, logic and extensive knowledge. I am a woman. I am eating. I am standing. I am thinking. Which of these statements is the most probable? Because they do not understand the content they generate, such systems are often called « stochastic parrots ». It is estimated that between 50 and 75% of newsrooms and journalists worldwide use generative AI. It is important to remember that ChatGPT has only been available for a year and has seen rapid and widespread adoption. It is not the only system available but perhaps the most popular. It can be used to perform everyday tasks already performed by AI systems. Still, the possibilities go beyond this, as it can be used as an assistant to generate ideas, angles, headlines, or rewrite content. Like any other AI system, generative AI technology relies on large amounts of data. The accessibility of the tools and their ease of use have enabled a democratisation of access to AI. That means that even small newsrooms can now afford to automate part of their processes.
6.
Defining data quality means first defining what information quality is. This is not an easy task as it is based on objective and subjective factors that relate to the credibility of the news. Scholars have provided different elements to understand what the concept of information quality refers to. According to Diakopoulos, it encompasses the dimensions in which journalists are concerned with trustworthy content: accuracy, comprehensibility, timeliness, reliability and validity. Sundar defined quality as a news story’s degree or level of overall excellence. It is an assessment of the goodness of a communication message. Clerwall emphasised that it consists of a general but somewhat vague notion of ‘that was a good story’ and that this subjective evaluation also depends on individual socio-professional backgrounds. Schweiger identified six dimensions to define quality: diversity, relevance, accuracy, understandability, impartiality and ethics.
7.
Data quality is equally difficult to define. It consists of a multidimensional concept that refers to formal and empirical indicators traditionally associated with the data’s accuracy, validity, completeness, consistency, uniqueness and timeliness. An interesting approach has been developed by Shank, based on the idea of approaching data quality from a semiotic point of view: the syntactic and semantic levels refer to truth, consistent structure and accurate meaning; the pragmatic level refers to fairness and usability; and the social level implies transparency and shared knowledge.
Given that a total data quality approach is not possible, especially in the context of empirical data that is likely to evolve, it is generally agreed that quality data refers to data that adapts to its final use. In journalism, it relates to the relevance of the use of the data, its completeness, accuracy and reliability. It implies the development of specific policies in terms of management, design, analysis, quality control, storage and presentation. These aspects can be addressed within the news media organisation if in-house solutions are developed by data or computer scientists or in collaboration with the newsroom. However, using external services in-the-shelf solutions means trusting the provider and the data it relies on.
8.
In data and computer science, there is a widely acknowledged principle: Garbage in, Garbage out. That means that the data quality in the input significantly impacts the output. Inaccurate or biased data can result in flawed machine-generated content, underscoring the importance of maintaining a high data quality level over time. Data quality issues are not trivial and have become more complex to tackle as the complexity of AI systems increases. Good data quality is not only about ensuring the accuracy and reliability of the outcomes. It is also a matter of trusting the systems and their products. The relationship between AI systems and end-users is also based on trust to favour adoption and integration within workflows. However, this is not as simple to tackle, depending on the technology used and whether it results from in-house development.
Nevertheless, issues related to data quality are often overlooked in journalism, including in classical data journalism activities, where it is often assumed that all data stored in a database can be used without being questioned. Considering the regular calls to infuse AI systems with journalistic values, it should start with data quality, even if it is only a part of the equation.
9.
The problem with AI is that it makes data quality issues much more complex to tackle, as they relate to an iterative process that includes data collection, pre-processing, validation, and training. The performance of an AI-based system depends on the algorithms used and their settings. Still, it also depends on the intrinsic characteristics of the data in terms of volume and completeness. In some cases, some tasks will require working with labelled data. This labelling process can be problematic because it may be carried out by low-paid workers whose expertise we don’t know. In the case of ChatGPT, African workers were hired to make the system less toxic or violent in its answers. AI systems need data to work. They need a lot of data. Hence, another temptation is to use all available data without assessing the source’s reliability. In automated fact-checking, for example, it is not uncommon to see systems that rely on Wikipedia, which cannot be considered a primary source in journalism because of its potential biases and errors, such as any other user-generated content.
10.
The ChatGPT case is one of the most problematic from the data quality perspective. Open AI does not reveal much about how ChatGPT was built and trained. According to an investigation published by the Washington Post, Wikipedia pages are the second most important source used by the system. ChatGPT’s training data also includes articles from news websites, books by scientific or fictional authors, and content generated by blog users and forums. The Washington Post also reported that ChatGPT was trained on data from religious platforms and far-right websites, raising concerns about potentially biased results.
Furthermore, most content used to train ChatGPT was copyrighted, but the company scraped the data without asking for consent or compensation. Private data are also suspected to be a part of the training datasets. As a result, ChatGPT cannot be considered ethical, even though it is widely used in newsrooms.
11.
ChatGPT is also notorious for generating inaccurate or false content, a phenomenon often called « artificial hallucination ». This involves adding information or creating realistic experiences that do not correspond to real-world input, representing semantic noise such as omissions, contradictions and invented content. This problem is widespread in Large Language Models (LLMs) due to their reliance on large datasets. The errors in ChatGPT are also attributed to the quality of the unsupervised training data, which includes challenges such as user-generated content (UGC) and biased data. The black-box nature of the system also contributes to its failures, making it difficult to track and fix these problems. The use of the term ‘hallucination’ has been criticised in this context, as it is seen as inappropriate to define bugs and fabricated responses. Of course, it is not cognitive hallucinations. ChatGPT never took LSD. Therefore, it was suggested that fictionalisation, extrapolation or even computational errors should be used instead.
12.
If data is biased, unfair, or inaccurate, it will lead to biased, unfair, or inaccurate outcomes. AI-based systems can serve as valuable news-gathering, production, and dissemination tools. At the same time, their use poses several risks that require careful consideration. First, these systems should be seen as a means rather than an end. Although these technologies can improve productivity and access to information, they are tools designed, trained, and used by humans. Only humans can mitigate the risks of potential negative impacts on information quality.
AI systems are also characterised by their dual nature, as they can be used to both inform and disinform. With generative AI technologies, creating fake news to manipulate public opinion has never been easy and quick. Hundreds of counterfeit websites are already powered by AI, with fake authors, images and content. At the same time, AI-based tools are also used to debunk manipulated content. There is room for improvement in these tools because technology evolves so fast, and results are more and more difficult to distinguish from reality. Yes, there are machine-generated detectors, but they are not working very well, creating false positives and negatives. However, what’s the point of knowing if the content was machine-generated or not, as it is not an indicator of the reliability of the content? In France, for instance, a news publisher has just launched a magazine whose content is 99% generated.
Therefore, clear information for the audience and realistic ethical guidelines are needed to avoid confusion, which can lead to behaviour that mistrusts AI results, whether providing non-manipulated news.
13.
It would have been unfair not to explain how AI tools can help improve the quality of information. All journalists are already using AI technology. They use AI-powered search engines like Google to gather information, check facts and research stories. They use machine translation services to access and interpret data in different languages. They use spell checkers to improve the quality of their writing. They also use automated transcription for their interviews. AI technology is everywhere. The possibilities to assist or augment journalists in their daily routines are numerous. They can automatically collect large amounts of data and create alerts on ‘hot’ topics. They can be used to investigate massive leaks such as the Panama Papers or, more recently, the NarcoLeaks. They can help make sense of vast amounts of data, manage all kinds of archives and facilitate information retrieval. The Finnish broadcaster Yle uses AI to promote diversity, for example, by translating Finnish content into Ukrainian to ensure that refugees receive accurate and fair information about the ongoing war. There are so many possibilities.
14.
The risk-based approach underpinning the AI Act approved a few days ago, can be mobilised to understand to what extent AI is likely to harm journalism or not. This approach is reflected in the recommendations of the French Press Council, the CDJM. The advantage of such an approach is that it distinguishes between tools that have the potential to cause harm and those that do not. Low-risk uses (e.g., spell-checking) have minimal impact on information and do not require audience notification. Moderate-risk uses, which are the responsibility of editors and journalists, may impact published information and must be explicitly disclosed to the public. They relate to the creation and production of content (e.g. audio synthesis, factual summaries, machine translation and automated reporting). Any generated content must be labelled as such, the data source must be acknowledged, and the journalist must publish the instructions for generating the content (or the prompts used in the case of generative AI). Finally, prohibited uses include generated content whose realism is likely to mislead audiences or present information contrary to the facts.
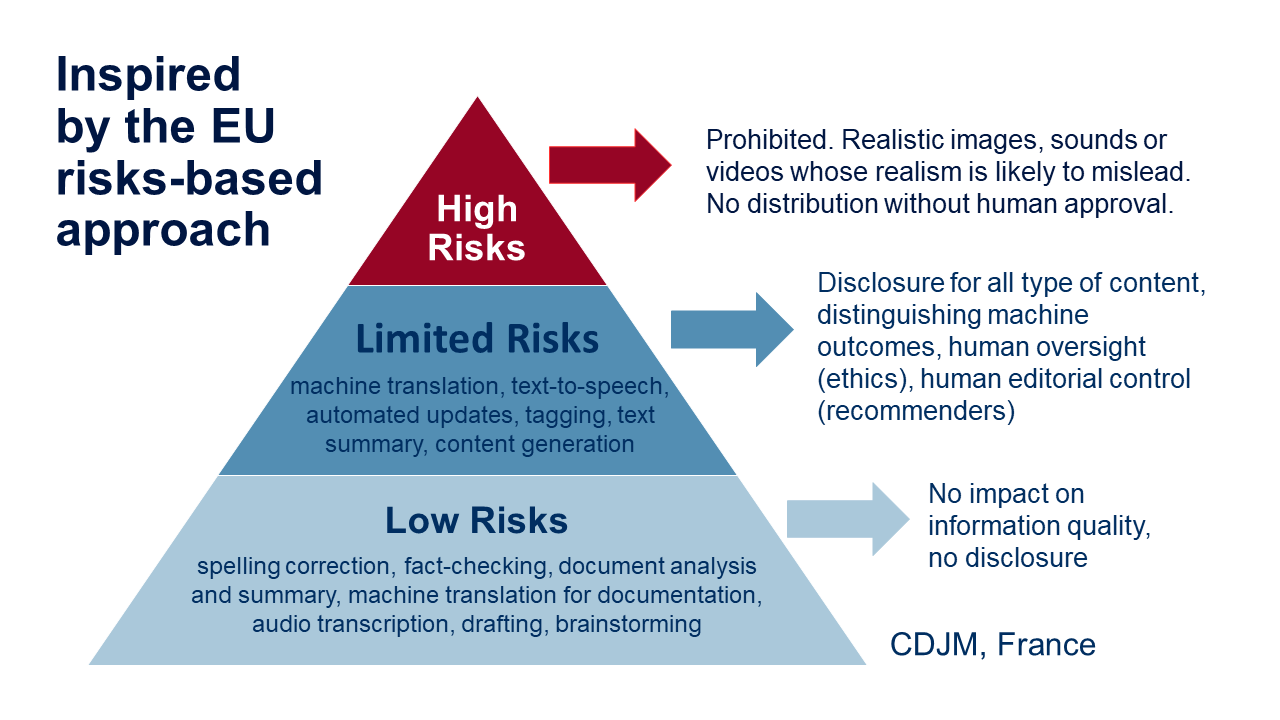
15.
At the University of Bergen, we wanted to understand how news and journalism organisations framed responsible uses of AI technology. Therefore, we analysed thirty-four texts, including two codes of ethics, fourteen guidelines, five position papers, ten general principles and three recommendations published by European news media organisations and self-regulatory bodies. Our form is currently under a peer review process. Since it was submitted, we identified nine new guidelines or recommendations. It illustrates the stressed need for clear frameworks not only for journalists but also for the audiences. The sharing of common principles is essential to avoid a proliferation of recommendations or guidelines that are likely to be contradictory.
Our analysis found a general acceptance of considering AI applications as an integral part of the editorial process. Transparency and the integration of a human-in-the-loop approach underline the importance of maintaining human control and oversight throughout the creation of AI-generated content. Recognising the importance of trusted data and the need for human management, the guidelines emphasise that final decision-making authority rests with humans, reinforcing the principle of human oversight in AI applications. The guidelines also uniformly support the disclosure of AI-generated content, emphasising the need for clarity about its provenance. In addition, informed consent is a critical consideration in news personalisation. Maintaining diversity in content creation and technological experimentation is encouraged, reflecting a commitment to promoting inclusivity and avoiding bias.
16.
In Europe, only two ethical codes of journalism include specific rules related to using artificial intelligence. In Flanders, de Raad voor de journalistiek introduced a new article 12 this year, which states that journalists are transparent about their approach and way of working. This transparency includes a clear disclosure of generated news content and recommender systems. Editors are responsible for editorial choices and the application and implementation of ethical principles regarding the system developers. The other code updated this year was published by Impress in the UK. The legend states that journalists should ensure the accuracy of any AI-generated content, considering that AI is also used for creating and distributing false content. The human oversight is required in any case. The code also states that transparency on the use of AI is needed, including a clear labelling of algorithmically recommended content.
17.
Transparency is critical to promoting credibility, accountability, responsibility, trust, and honesty. However, equating clarity with responsibility raises concerns about its societal implications, necessitating a distinction between journalistic roles and individual responsibilities. Transparency faces challenges in addressing the intricacies of decision-making processes within AI systems. The association of transparency with unbiased methods clashes with AI’s complex and biased nature. Even when transparency is accompanied by explainability, the results may lack trustworthiness due to a lack of contextual understanding, leading to user misinterpretation. Maintaining openness and explainability also requires continuous user education on complex and evolving systems. In addition, even if journalists are transparent about their use of AI, it doesn’t guarantee explainability. Journalism is not entirely transparent neither, as parts of the editorial decision-making process remain hidden from audiences, that’s the black-box of journalism.
18.
News media organisations are generally aware of the possibility of artificial hallucinations. If most of them promote experimenting with generative AI, they consider that it should be made carefully, considering the limitations of the tools. ANP emphasises that it is complex to guarantee the reliability of the facts presented as factual. De Volskrant underlines that it is difficult to know what data the system was trained on. STT in Finland also underscores the uncertainty related to the sources used in AI systems. For Wired, generative AI can generate false leads and boring ideas.
19.
Despite the importance of data quality, only a few texts focused on this specific matter, which supposes developing robust data and AI literacy. The work done by the Catalan Press Council illustrates in-depth work led together by academics and professionals to make good practices. Several organisations also highlighted the importance of involving journalists in the design or decision-making process for implementing AI in newsrooms. While this can be seen as a good step, it must rely on robust data and AI literacy. In our research, we found that this aspect was largely overlooked. We also found that very few organisations considered the benefits of working closely with academia, where considerable knowledge is being developed from an interdisciplinary perspective.
20.
AI in journalism is not just the domain of journalists, as it involves data and computer scientists who are very specialised in their field. However, they are not trained in journalism and are often left out of the discussion. It supposes being able to understand each other as the meanings of some concepts, such as accuracy, objectivity, and transparency, are likely to differ from one social world to another. Interestingly, the BBC first published guidelines for developers rather than journalists, stating that machine learning tools must be consistent with the values of the public service broadcaster. The BBC’s principles emphasise that the responsibility for AI lies with those who build the system. Developers must, therefore, be aware of the consequences of their decisions regarding personalised content. Like journalism, technology is human and only part of the process. Responsible engineering was also at the heart of the guidelines published by Bayerischer Rundfunk (BR), which worked with start-ups and universities to develop an interdisciplinary perspective.
Fostering more collaborations and more interdisciplinary is a critical key to moving forward in these continuous environments and promoting ethical and responsible use on either side of the system.
21.
As I explained at the beginning of my talk, developing robust AI literacy is also needed for journalists to investigate algorithmically driven societies. AI literacy enables journalists to navigate complex technical concepts, ask informed questions, and interpret data, fostering a more accurate and nuanced portrayal of these technologies that permeate our everyday lives. It is about the democratic role of journalism. Four years ago, it was disclosed that the Dutch tax authorities employed a self-learning algorithm to identify potential fraud in childcare benefits. Families were penalised based on the system’s risk indicators, resulting in numerous households, particularly those with lower incomes or from ethnic minorities, facing financial hardship due to substantial debts to the tax agency. The use of the algorithm led to widespread consequences, pushing tens of thousands of families into poverty based on mere suspicions of fraud. More recently, the newspaper Le Monde revealed that French authorities used an algorithm to detect social fraud in France. Here, the system discriminated against the most vulnerable people. Being better equipped to understand the possibilities, the limits, and the grey areas of AI-driven systems is full of benefits. The society has already evolved, and it will continue to transform. Journalists must take care of it. It is also a matter of information quality.