Topic modeling is a statistical model for discovering topics or concepts in documents (Schweinberger, 2021). It fits into the larger framework of distant reading, a concept introduced by Moretti (2013), which refers to the quantitative analysis of textual data.
The Latent Dirichlet Allocation (LDA) algorithm is probably the most popular for topic modeling of a corpus of textual documents. In particular, it makes it possible to evaluate the distribution of topics within the corpus. It can be used both for classification and documents comparisons.
The LDA (Blei, 2003) is part of the family of unsupervised learning algorithms. Thus, it does not require any human annotation activity. It is a probabilistic model based on a three-level hierarchical Bayesian model. Each collection element is modeled as a finite mixture on an underlying set of subjects. Each subject is, in turn, modeled as an infinite mixture on an underlying set of subject probabilities’ (Blei, Ng and Jordan, 2003). Empirically, probabilistic modeling generally leads to good results and gives rise to significant semantic decompositions.
The LDA algorithm is included in the R topicmodels package, and there are different ways to program it. Results may differ depending on the settings used and the number of topics desired (k). One uses Gibbs sampling, an algorithm that successively samples converging conditional distributions. It is arguably the most efficient method in that it produces more variety in the modeling (Chang et al., 2009).
Topic Modeling 1 (LDA, Gibbs)
Topic Modeling 2 (LDA)
The results obtained with the LDA algorithm refer to the topics of the booster (third dose of vaccine), health measures (lockdown and vaccine pass), side effects of the vaccine, the clinical trial phase of vaccines, the spread of the omicron variant, the unvaccinated or ‘anti-vax’ people, the mediatic discourses about the health crisis, the scientific discourses, and childhood vaccination. While these topics correlate with those identified during the lexical analysis (frequency of terms, bigrams, and trigrams), they also uncover trends that were not observed until now.
This topic modeling is confirmed by examining the Correlated Topic Model (CTM), a hierarchical document collection model. Very resource-intensive, this algorithm is limited to small models (He et al., 2017). However, there is an advantage in modeling the correlation of subjects. And, in some cases, it gives a better fit than the LDA (Blei & Lafferty, 2006), as it is observed in the third model where the concepts of doubt and trust (towards politicians, vaccines, pharmaceutical companies, science, and the media) appear in the background.
Topic Modeling 3 (CTM)
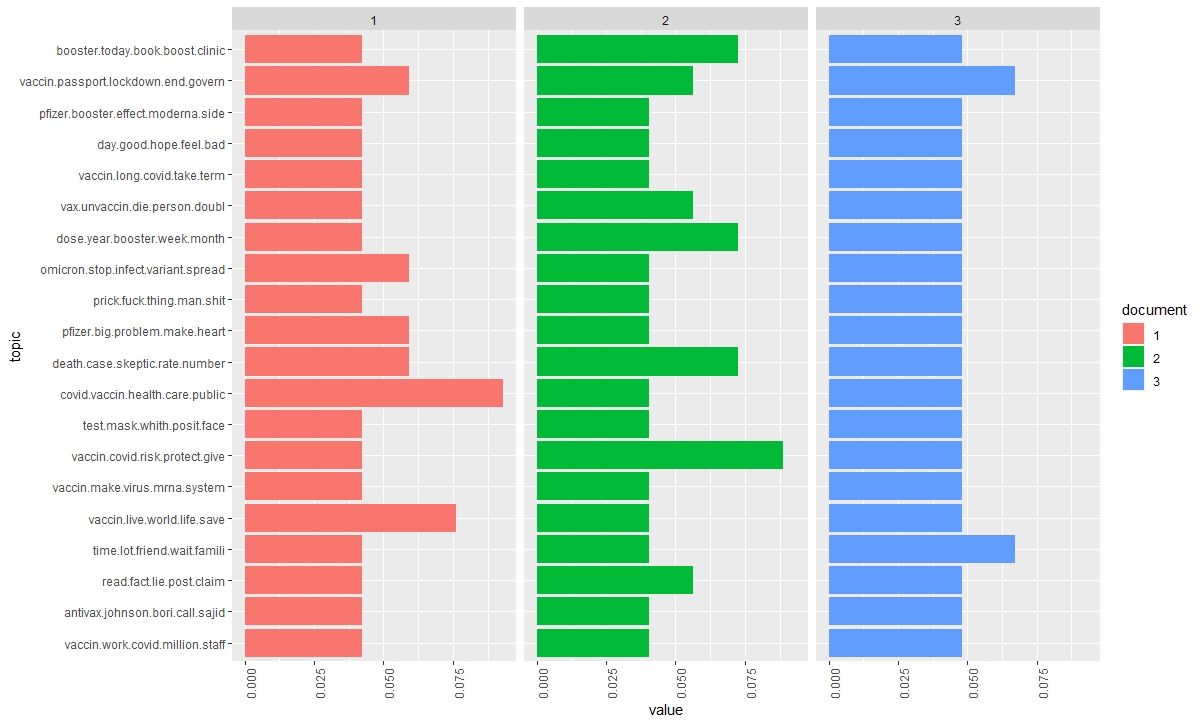
This analysis of the topic modeling of the ‘vaccines’ corpus confirms the previous analyses: the crisis is about health and has substantial political and societal implications. The situation divides people between pro and anti-vaccines, which are criticized for their stupidity, idiocy, and conspiracy. Therefore, the question of the polarization of discourse is confirmed at each level of analysis of this first corpus of tweets (to be continued).
References
Blei, D. M., Ng, A. Y., and Jordan, M. I. (2003). Latent Dirichlet Allocation. Journal of Machine Learning Research, 3:993-1022.
Blei, D., and Lafferty, J. (2006). Correlated topic models. Advances in neural information processing systems, 18:147.
Chang, J., Gerrish, S., Wang, C., Boyd-Graber, J. L., and Blei, D. M. (2009). Reading tea leaves: How humans interpret topic models. In Advances in neural information processing systems (pp. 288-296).
He, J., Hu, Z., Berg-Kirkpatrick, T., Huang, Y., and Xing, E. P. (2017, August). Efficient correlated topic modeling with topic embedding. In Proceedings of the 23rd ACM SIGKDD International Conference on Knowledge Discovery and Data Mining (pp. 225-233).
Hornik, K., and Grün, B. (2011). topicmodels: An R package for fitting topic models. Journal of statistical software, 40(13):1-30.
Moretti, F. (2013). Distant reading. Verso Books.
Schweinberger, M. (2021). Topic Modeling with R. Brisbane: The University of Queensland. URL: https://slcladal.github.io/topicmodels.html (Version 2021.11.24).
Silge, J., and David, R. (2017). Text Mining with R: A Tidy Approach. O’Reilly Media, Inc.
Resources
- Latent Dirichlet Allocation Using Gibbs Sampling: https://ethen8181.github.io/machine-learning/clustering_old/topic_model/LDA.html
- Topic modeling using Latent Dirichlet Allocation(LDA) and Gibbs Sampling explained: https://medium.com/analytics-vidhya/topic-modeling-using-lda-and-gibbs-sampling-explained-49d49b3d1045
- Topic Modeling in R With tidytext and textmineR Package (Latent Dirichlet Allocation): https://medium.com/swlh/topic-modeling-in-r-with-tidytext-and-textminer-package-latent-dirichlet-allocation-764f4483be73
- Topic modeling: https://www.rtextminer.com/articles/c_topic_modeling.html
- Topic Modeling of Surah Al-Kahfi: https://rpubs.com/azmanH/716181
- Intuitive Guide to Correlated Topic Models: https://towardsdatascience.com/intuitive-guide-to-correlated-topic-models-76d5baef03d3
- Package R ‘topicmodels’: https://cran.r-project.org/web/packages/topicmodels/topicmodels.pdf
- Package R ‘tm’: https://cran.r-project.org/web/packages/tm/vignettes/tm.pdf